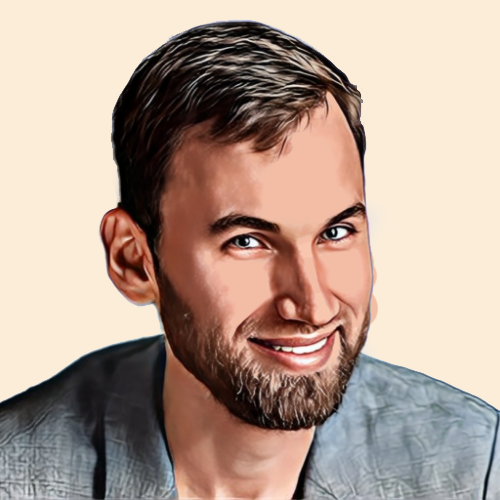
In a world where artificial intelligence (AI) is increasingly permeating the financial sector, institutional investors are understandably asking the question: how much does ChatGPT really know about finance? The answer is more nuanced than many might expect.
A recent study (published in the Financial Analysts Journal) examined over 10,000 AI-generated responses to official financial exam questions (SIE, Series 6, 7, 65, 66). The outcome? ChatGPT and other large language models (LLMs) show remarkable potential—but also clear limitations.
ChatGPT (almost) passes the test
Among the freely available models, ChatGPT-3.5 scored just over 63 percent correct on multiple-choice questions—not bad, but not good enough to rely on non-human experts. ChatGPT-4, the paid version, performed significantly better with 84.5 percent correct answers. For context, these are entry-level exams for financial professionals.
But it’s not just about picking the right answer. Researchers also evaluated whether the model could justify its choices the way a human expert would. Here, LLaMA stood out with the highest semantic similarity in explanations, though it lagged in factual accuracy. Once again, ChatGPT-4 struck the best balance between correctness and explanation.
Executing tasks
More important than exam scores is how well these models translate to real-world tasks within an investment organization. By mapping exam questions to 51 specific finance-related tasks, the authors discovered that the strength of AI language models depends more on the task than the job title.
Tasks like monitoring markets or explaining investment concepts scored high in accuracy. Think questions like: “What happens to bond prices when interest rates rise?” or “What’s the difference between ETFs and mutual funds?” ChatGPT handles these well.
However, for more complex, context-dependent assignments—such as analyzing client profiles or tax situations—the model performs at or below human level. The risk of incorrect interpretation is too high to deploy AI without oversight.
The role of fine-tuning
Institutions can improve model performance through fine-tuning, feeding the model with specific training data. This significantly increased the similarity to human explanations, though factual accuracy still depended on the wording of the question and parameters used.
Access via API, with control over parameters like randomness or verbosity, proved essential. Higher accuracy requires lower randomness and more concise output. In other words, anyone looking to use ChatGPT as a reliable colleague needs to think carefully about how the model is deployed.
What this means for institutional investors
LLMs are not a short-term replacement for investment professionals, but they are a powerful complement. Consider applications like:
- Rapid screening of frequently asked questions
- First drafts of investment reports
- Educational support for junior staff
- Summaries of regulations or market analysis
At the same time, the authors caution against premature use in client interactions, compliance-related cases, or strategy development. In these areas, human oversight is not a luxury—it’s a necessity.
Conclusion
ChatGPT is not the next Warren Buffett, but it’s no longer just a simple chatbot either. For institutional investors willing to use AI thoughtfully, task-specifically, and under supervision, the added value is clear. That said, it’s essential to understand the model’s limitations and remain critical about where and how it’s deployed. AI is not a replacement for expertise—it’s an enhancement of it.
Gertjan Verdickt is an Assistant Professor of Finance at the University of Auckland and a columnist for Investment Officer.