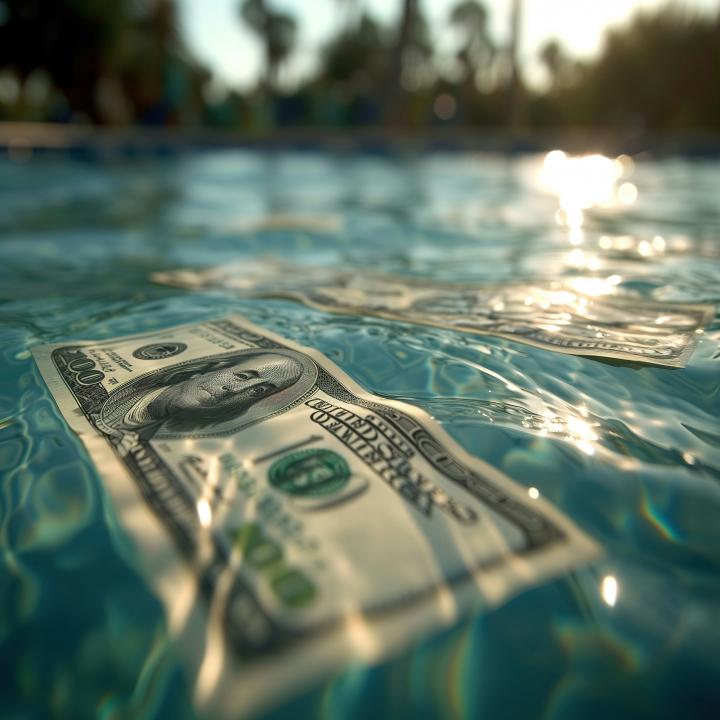
Artificial intelligence is fast becoming a game-changer in fixed-income investing, offering powerful new tools to understand and forecast bond market liquidity, an essential metric for active bond managers and institutional investors.
Traditionally, bond liquidity has been difficult to quantify. Trading in fixed income markets is less centralised and less frequent than in equities, with wide differences in issuance size, maturity, and credit quality. This makes it harder to assess how easily a bond can be bought or sold without affecting its price.
Christian Kronseder, CEO of Zurich-based fintech Allindex, puts it bluntly. “The liquidity of a bond is a tricky thing because you first have to find it.”
Hard to quantify
“Depending on the market, that can be very easy, or not. In large markets like the US or Europe, it’s manageable,” he explained. “But in smaller markets like Switzerland, if you don’t know the right trader, it can be a bad day. Liquidity is more of an idea than a fixed measure. Because it’s so hard to quantify, it’s not fully understood, which is why, considering the way most people approach it today, building liquidity-optimised portfolios is almost impossible.”
Yet that may be starting to change. Recent advances in artificial intelligence, especially in machine learning and deep learning, are opening new ways to analyse and forecast liquidity with a level of granularity and speed not previously possible. These developments are particularly relevant to wealth managers, multi-asset investors and fixed income desks who need to stay ahead of liquidity trends in both normal and stressed market conditions.
Next-generation forecasting
Allindex is one of a growing number of fintech firms applying cutting-edge AI to this long-standing problem. Using bond dealer data, its system draws on a wide range of inputs, from transaction size and bid-ask spreads to historical trading data and dealer pricing, and combines them to forecast liquidity up to two weeks in advance. This predictive capability can make a material difference to how investment strategies are constructed and executed, especially in high-yield or less liquid markets.
Random forests and XGBoost
The technology uses a blend of models. In tech-speak: a combination of two relatively simple AI models, known as ‘random forests‘ and ‘XGBoost’. The outcome then is combined again with a LLM model, which enables managers to question the data. This approach makes it possible to enhance the explainability, Kronseder told Investment Officer.
“We are using random forests and XGBoost to enhance the explainability. Combining a LLM with our models will help to explain in detail which decision was taken at what time,” he said.
Sophisticated financial data analysis tools often use models known as LSTMs, known as Long Short-Term Memory. Even more sophisticated is the use of convolutional neural networks, or CNNs, something with which Allindex is experimenting.
“Portfolios managers have to be able to explain their decisions.”
Christian Kronseder, Allindex
However, random forests and XGBoost are like smart decision-makers that work well with tables of numbers, even if some data is missing. They’re quick, easy to understand, and don’t need much cleanup. LSTMs and CNNs are better for things like speech or images but are slower and need more training and data.
“The advantage of the two simpler models is their explainability,’ Kronseder said. “LSTMs are generally applied as a deep learning model for time series. That is correct. The main problem is its explainability.”
“Why is that important? Portfolios managers have to be able to explain their decisions. Deep learning models are hard to explain. The two other models can be explained in detail.”
A key feature is the system’s ability to handle non-stationary markets, where conditions shift rapidly. Using a “sliding window” approach, the models update continuously based on recent developments, enabling more timely and responsive forecasts compared to static, backward-looking analysis.
From insight to strategy
This new approach to liquidity forecasting offers a range of practical benefits for fixed income investors. It enables real-time assessments of market liquidity, helps identify the most liquid bonds within a given investment universe, and provides historical liquidity benchmarks that support more accurate risk modelling. In addition, it delivers valuable estimates of transaction costs and facilitates liquidity stress testing and scenario planning, all of which contribute to more informed and resilient portfolio management.
For Kronseder, the ultimate aim is to integrate these capabilities into a broader portfolio optimisation framework. “We’re not stopping here,” he said. “We’d like to build a comprehensive solution that can help optimise portfolios and support smarter allocation decisions.”
The potential upside is significant. In volatile or fast-moving markets, access to real-time liquidity signals can inform better trade execution and reduce slippage. For multi-asset managers, understanding bond liquidity in the context of wider portfolio construction allows for more dynamic rebalancing and improved resilience under stress.